Reality AI Lab pushes the boundaries of artificial intelligence, creating hyperrealistic simulations with profound implications across various sectors. We delve into the core technologies driving this innovation, from advanced machine learning algorithms to cutting-edge rendering techniques, exploring how these tools are shaping the future of healthcare, engineering, and urban planning. This exploration unveils the potential, the challenges, and the ethical considerations inherent in building truly realistic AI environments.
Imagine a world where surgeons practice complex procedures on virtual patients, engineers test designs in flawless digital twins, and urban planners model entire cities to optimize resource allocation and predict future growth. This isn’t science fiction; it’s the promise of Reality AI Lab. By harnessing the power of AI, we are building tools to solve some of humanity’s most pressing problems and unlock unprecedented opportunities for innovation.
Introduction to Reality AI Lab
Reality AI Lab is a pioneering research facility dedicated to bridging the gap between artificial intelligence and the real world. Our mission is to develop and deploy cutting-edge AI technologies that seamlessly integrate with and enhance our daily lives, focusing on applications that are both beneficial and ethically sound. We aim to achieve this through collaborative research, open innovation, and a commitment to responsible AI development.The research conducted at Reality AI Lab promises to have a significant impact across various sectors.
Our advancements in AI-driven perception, prediction, and decision-making will revolutionize industries ranging from healthcare and manufacturing to transportation and environmental monitoring. For example, our work on autonomous vehicle navigation will improve road safety and efficiency, while our research in medical imaging analysis will lead to earlier and more accurate disease diagnosis.
Technological Infrastructure
The Reality AI Lab relies on a robust and sophisticated technological infrastructure to support its ambitious research goals. This includes high-performance computing clusters capable of processing massive datasets, advanced sensor networks for data acquisition in real-world environments, and a secure data storage and management system to ensure data integrity and privacy. Furthermore, we utilize specialized software tools and development platforms tailored for AI research, including machine learning frameworks, simulation environments, and data visualization tools.
Access to a diverse range of datasets, both publicly available and proprietary, is also crucial to our work. Our infrastructure also incorporates dedicated spaces for robotic experimentation and testing, providing a controlled environment for evaluating the performance and safety of our AI systems. This multifaceted approach ensures we can conduct research across a wide range of AI applications, from the development of sophisticated algorithms to the deployment and testing of real-world systems.
Core Technologies of Reality AI Lab
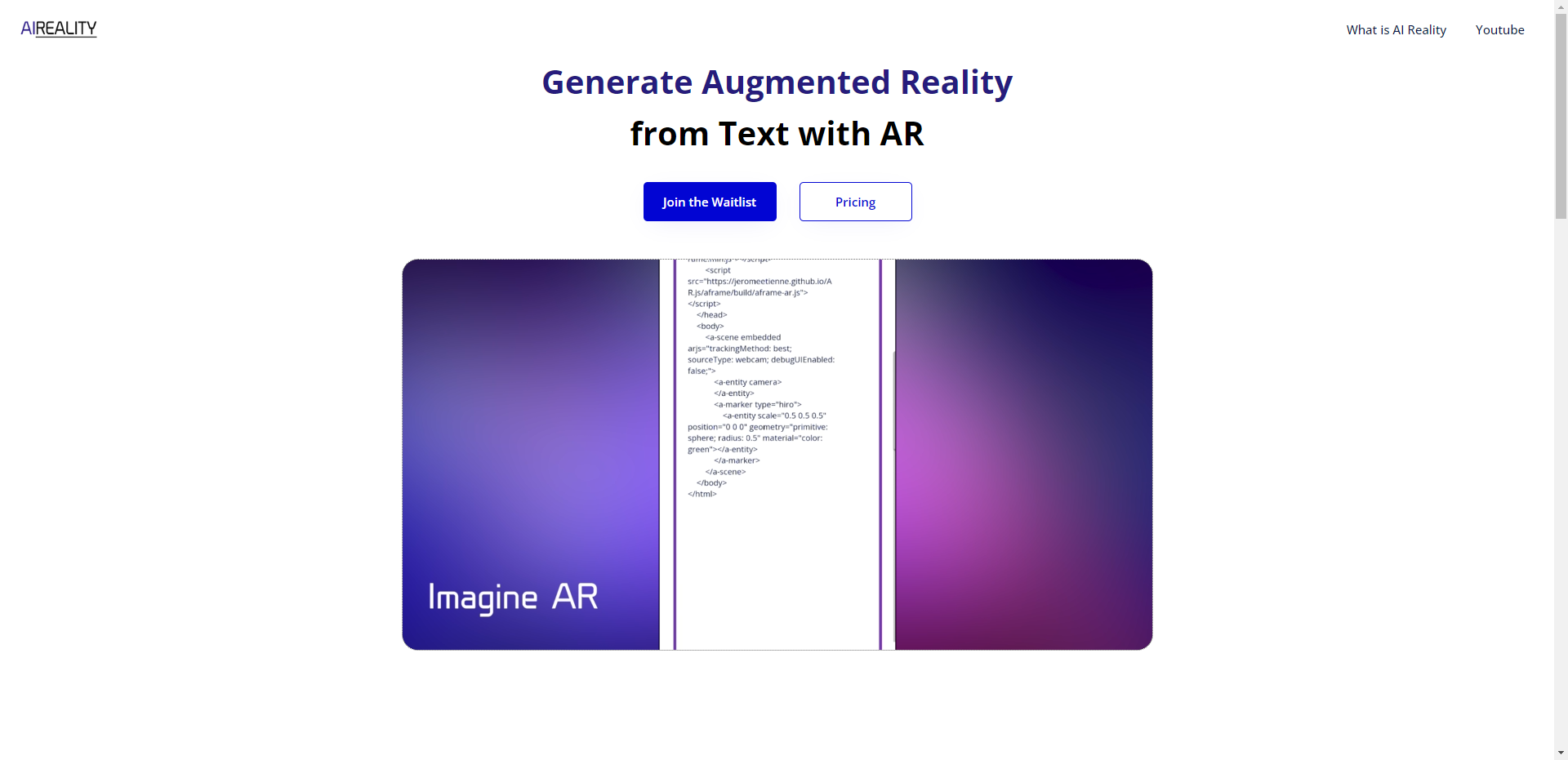
A Reality AI Lab leverages a potent combination of artificial intelligence and computer graphics to create incredibly realistic and interactive simulations. These simulations are not just visually appealing; they serve as powerful tools for research, development, and training across various fields. The core technologies underpinning this capability are diverse and constantly evolving.The foundation of any Reality AI Lab lies in the synergy between several key AI technologies.
These technologies work together to achieve a high degree of realism and interactivity within the simulated environments.
Key AI Technologies Employed
The core technologies driving realistic simulations in a Reality AI Lab include deep learning, particularly for computer vision and natural language processing, alongside advanced physics engines and procedural generation techniques. Deep learning models are trained on vast datasets of real-world data to learn the intricate patterns and nuances of reality, enabling them to generate highly realistic visuals, sounds, and even behaviors.
Physics engines ensure that objects interact realistically within the simulation, obeying the laws of physics, while procedural generation algorithms create vast and diverse environments without manual intervention. For instance, a deep learning model might be trained on thousands of images of forests to generate realistic tree models, while a physics engine would simulate the way branches sway in the wind.
Natural language processing allows for realistic interaction with the simulated environment through voice commands and conversational AI.
Approaches to Creating Realistic Simulations
Several approaches exist for generating realistic simulations, each with its strengths and weaknesses. One common approach is physics-based simulation, where every element’s behavior is dictated by physics equations. This method provides high accuracy but can be computationally expensive, limiting the scale and complexity of the simulated environment. Alternatively, data-driven simulation leverages machine learning models trained on real-world data to predict and generate realistic behaviors.
This approach can handle larger, more complex environments but might sacrifice some level of physical accuracy for speed and scalability. A hybrid approach, combining physics-based and data-driven methods, often provides the best balance between accuracy, realism, and computational efficiency. For example, a simulation of a city might use physics-based models for individual vehicle movements but data-driven models to predict overall traffic patterns.
Hypothetical Project Workflow
A typical project within a Reality AI Lab follows a structured workflow. It begins with data acquisition, where relevant data is gathered – this could involve collecting images, videos, sensor data, or even textual descriptions of the environment being simulated. This data is then pre-processed and cleaned to ensure quality and consistency. The next stage involves model training, where AI models are trained using the prepared data to learn the characteristics of the target environment.
These models are then integrated into a simulation engine, often a game engine adapted for AI-driven interactions. Following this, the simulation is rigorously tested and refined, with iterative adjustments made to improve realism and performance. Finally, the completed simulation is deployed, ready for use in research, training, or other applications. For instance, a project aiming to simulate a wildfire might involve acquiring satellite imagery and meteorological data, training models to predict fire spread, and integrating these models into a game engine to visualize the wildfire’s progression.
Applications of Reality AI Lab Research
Reality AI Lab’s research, focusing on advanced AI and its interaction with the real world, has far-reaching implications across numerous sectors. Its core technologies, including advanced sensor fusion, real-time processing, and robust AI models, translate into practical applications with the potential to revolutionize how we interact with our environment and each other. The following sections explore some key application areas and their associated ethical considerations.
Healthcare Applications of Reality AI Lab Research
Reality AI Lab’s research offers significant potential for improving healthcare diagnostics, treatment, and patient care. For instance, real-time analysis of medical images using AI could lead to faster and more accurate diagnoses of diseases like cancer. Imagine a system that instantly analyzes a CT scan, highlighting suspicious areas with pinpoint accuracy and providing the radiologist with crucial data for a more informed decision.
Furthermore, AI-powered robotic surgery could enhance precision and minimize invasiveness, leading to faster recovery times and reduced complications. Wearable sensors integrated with AI algorithms could continuously monitor patients’ vital signs, alerting medical professionals to potential problems before they become critical. This proactive approach to healthcare could save lives and improve overall health outcomes.
Engineering and Design Applications of Reality AI Lab Research
The applications in engineering and design are equally transformative. Reality AI Lab’s technology could optimize building designs for energy efficiency by simulating various environmental conditions and predicting energy consumption patterns. This would lead to more sustainable and cost-effective buildings. In manufacturing, AI-powered quality control systems could detect defects in real-time, improving product quality and reducing waste. Autonomous robots guided by Reality AI Lab’s algorithms could perform complex tasks in hazardous environments, such as inspecting pipelines or repairing damaged infrastructure, enhancing worker safety and efficiency.
Moreover, the creation of realistic simulations using AI could revolutionize product design, allowing engineers to test and refine designs virtually before physical prototypes are even built.
Ethical Considerations and Societal Impact of Reality AI Lab Research
The development and deployment of AI technologies like those developed by Reality AI Lab raise important ethical considerations. Bias in algorithms, data privacy concerns, and the potential for job displacement are all significant issues that require careful consideration. Responsible development and deployment of these technologies necessitate transparency, accountability, and a commitment to fairness and equity. The societal impact could be profound, potentially leading to significant improvements in healthcare, safety, and efficiency, but also posing risks that need careful management.
For example, the use of AI in healthcare could exacerbate existing health disparities if not carefully designed and implemented to address equity issues.
Application | Benefits | Risks | Mitigation Strategies |
---|---|---|---|
AI-powered medical diagnosis | Faster and more accurate diagnoses, improved patient outcomes | Algorithmic bias, data privacy concerns, potential for misdiagnosis | Rigorous testing and validation, data anonymization, human oversight |
AI-guided robotic surgery | Increased precision, reduced invasiveness, faster recovery times | System malfunctions, high initial cost, potential for job displacement | Redundant safety systems, phased implementation, retraining programs |
AI-powered autonomous vehicles | Improved traffic flow, reduced accidents, increased accessibility | Safety concerns, ethical dilemmas in accident scenarios, job displacement | Thorough testing and validation, clear ethical guidelines, social safety nets |
Challenges and Future Directions for Reality AI Lab
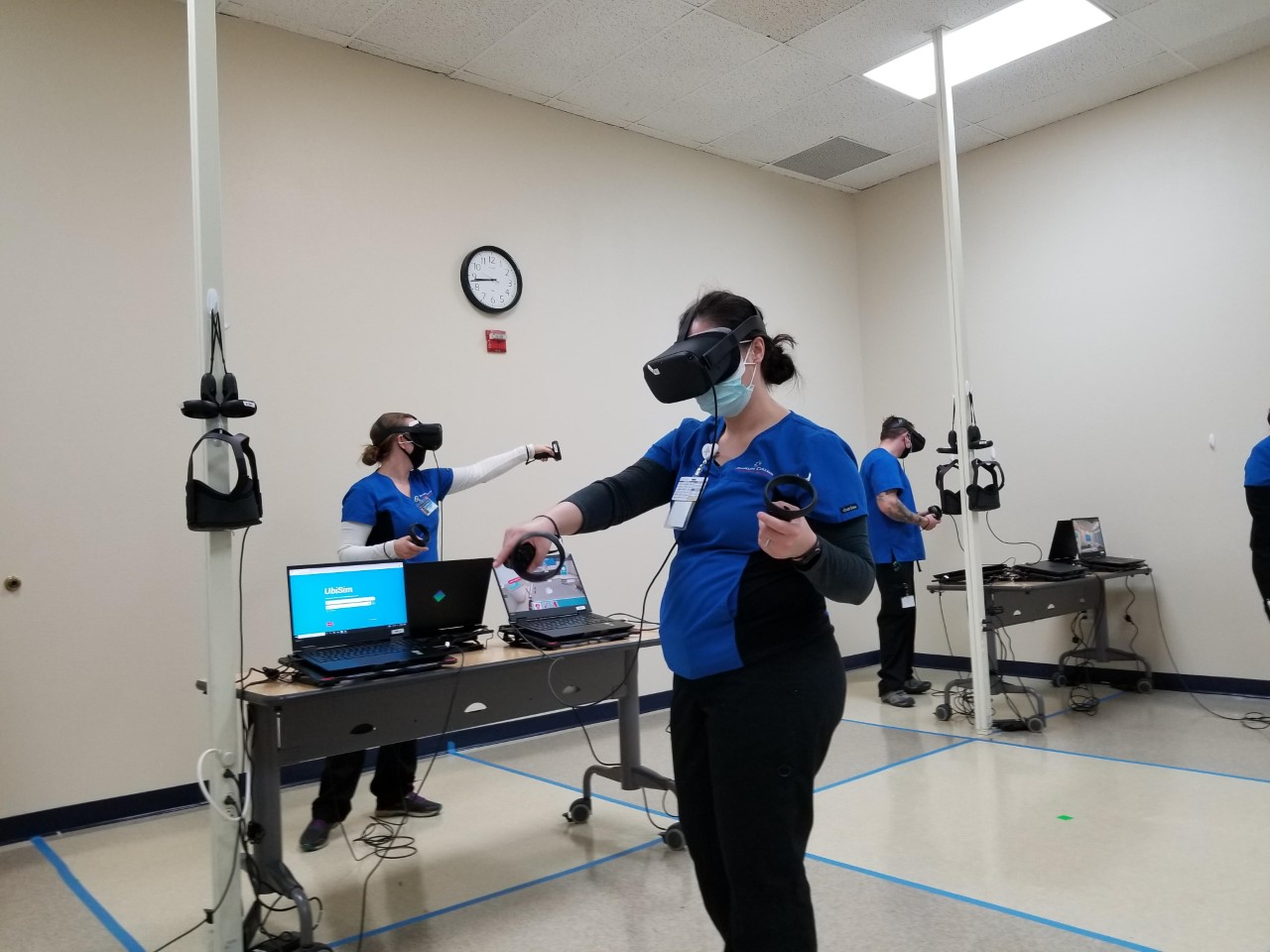
Building a truly realistic and interactive AI environment presents significant hurdles. The Reality AI Lab faces a complex interplay of technological, computational, and collaborative challenges that will shape its future trajectory. Overcoming these obstacles will not only advance the field of AI but also unlock transformative applications across various industries.
Technological Hurdles Facing Reality AI Labs
Developing truly realistic AI necessitates tackling several key technological limitations. High-fidelity rendering of complex environments demands immense computational power, often exceeding the capabilities of current hardware. Furthermore, creating believable AI agents requires sophisticated algorithms capable of handling diverse and unpredictable interactions. Accurate and efficient physics simulation, crucial for realistic interactions, remains a computationally intensive challenge. Finally, the creation of seamless and intuitive human-AI interfaces is a significant design and engineering hurdle.
Addressing these limitations will require breakthroughs in both hardware and software development. For example, the development of specialized AI chips designed for real-time rendering and physics simulation could significantly alleviate computational bottlenecks. Similarly, advancements in reinforcement learning and generative models could improve the realism and adaptability of AI agents.
Potential for Future Breakthroughs in Reality AI Lab Research
The potential for groundbreaking advancements within Reality AI Lab is considerable. Improvements in deep learning architectures could lead to more nuanced and human-like AI agents, capable of exhibiting complex emotions and behaviors. Advances in natural language processing (NLP) could enable more natural and intuitive communication between humans and AI within the simulated environment. The integration of advanced haptic feedback systems could further enhance the sense of immersion and realism, leading to more engaging and effective applications.
For instance, imagine surgeons practicing complex procedures in a fully realistic virtual operating room, benefiting from haptic feedback that replicates the feel of tissues and instruments. This level of realism could significantly improve surgical training and outcomes.
The Need for Interdisciplinary Collaboration within a Reality AI Lab
Success in Reality AI research demands a strong interdisciplinary approach. The creation of realistic AI environments requires expertise from computer scientists, engineers, psychologists, neuroscientists, and artists. Computer scientists provide the core algorithms and computational infrastructure. Engineers build the hardware and software systems. Psychologists and neuroscientists offer insights into human behavior and cognition, crucial for designing believable AI agents.
Artists contribute to the creation of visually compelling and immersive environments. This collaborative environment fosters innovation and ensures the development of realistic and ethically sound AI systems. For example, a collaborative effort between computer scientists specializing in physics simulation and neuroscientists studying human motor control could lead to more realistic and intuitive interactions within the simulated environment.
Potential Research Grants for Reality AI Lab
Securing funding is crucial for pushing the boundaries of Reality AI research. Several potential grant opportunities exist, each focusing on a specific aspect of the field:
- National Science Foundation (NSF)
– “Next Generation AI for Immersive Simulations”: This grant would focus on developing novel algorithms and hardware for creating highly realistic and interactive AI environments, addressing computational limitations and improving agent realism. - Defense Advanced Research Projects Agency (DARPA)
-“AI-Driven Synthetic Training Environments”: This grant would support the development of realistic AI-powered simulations for military training, focusing on creating believable adversaries and dynamic environments. - European Research Council (ERC)
-“Bridging the Gap Between Virtual and Real-World Interactions”: This grant would fund research aimed at improving the realism and intuitiveness of human-AI interaction within virtual environments, exploring novel haptic and sensory feedback technologies. - National Institutes of Health (NIH)
-“AI-Assisted Medical Training and Simulation”: This grant would focus on developing realistic AI-powered medical simulations for training purposes, enhancing the effectiveness of medical education and improving patient outcomes. For example, simulating complex surgical procedures with realistic haptic feedback.
Case Studies
Let’s dive into some hypothetical projects showcasing the potential of Reality AI Lab’s technology across diverse fields. These examples illustrate the power of realistic AI simulation in tackling complex real-world problems.
Reality AI Lab focuses on cutting-edge AI applications, and one fascinating area is how AI improves drone navigation and safety. For example, imagine the complexities of drone flight in a dense urban environment like Paris; check out this resource on the challenges and opportunities: drone in paris. Understanding these challenges helps Reality AI Lab develop robust AI systems for safer and more efficient drone operations in complex environments worldwide.
Realistic Human Behavior Simulation in a Crowded Urban Environment
This project aims to simulate realistic human behavior within a highly detailed 3D model of a bustling city center. The goal is to predict pedestrian movement patterns, identify potential bottlenecks, and optimize urban infrastructure for improved pedestrian flow and safety. We’d leverage advanced AI models trained on massive datasets of real-world pedestrian movement data, incorporating factors like time of day, weather conditions, and special events to generate highly accurate simulations.
The simulation would incorporate diverse agent behaviors, accounting for individual differences in walking speed, pathfinding strategies, and responses to obstacles. This project could provide invaluable insights for urban planners seeking to improve pedestrian safety and experience in densely populated areas. For instance, the model could pinpoint areas with high pedestrian congestion and suggest design modifications like widening sidewalks or adding pedestrian crossings to alleviate congestion and enhance safety.
Virtual Training Environment for Emergency Response Teams
This project focuses on creating immersive virtual training environments for emergency response teams, such as firefighters and paramedics. The training scenario involves a simulated building fire, complete with realistic smoke propagation, structural damage, and trapped civilians. Trainees navigate the virtual environment, utilizing virtual equipment to locate and rescue the civilians. The AI system dynamically adjusts the scenario’s complexity based on the trainees’ performance, providing personalized challenges and feedback.
Assessment methods would involve objective measures like time taken to complete tasks, successful rescues, and adherence to safety protocols, alongside subjective evaluations based on expert observation of decision-making and teamwork. This would provide a cost-effective and safe way to train emergency responders in high-stakes situations, improving their skills and preparedness for real-world emergencies. Think of it as a sophisticated flight simulator, but for emergency response scenarios.
Urban Planning and Development using AI-Powered Simulation
This project applies Reality AI Lab’s technology to optimize urban planning and development decisions. Data sources would include high-resolution satellite imagery, census data, traffic patterns, and environmental data. The model would simulate the impact of various development scenarios, such as the construction of new buildings, roads, or parks, on factors like traffic congestion, air quality, and property values.
Modelling techniques would involve agent-based modelling, coupled with machine learning algorithms to predict the effects of different development options. For example, the simulation could predict the impact of building a new highway on traffic flow in surrounding areas, allowing urban planners to assess potential bottlenecks and optimize road design. This approach enables data-driven decision-making, leading to more sustainable and efficient urban development.
The project could also incorporate predictive models for future population growth and economic activity to anticipate long-term impacts of development decisions.
Visual Representation of Reality AI Lab Concepts
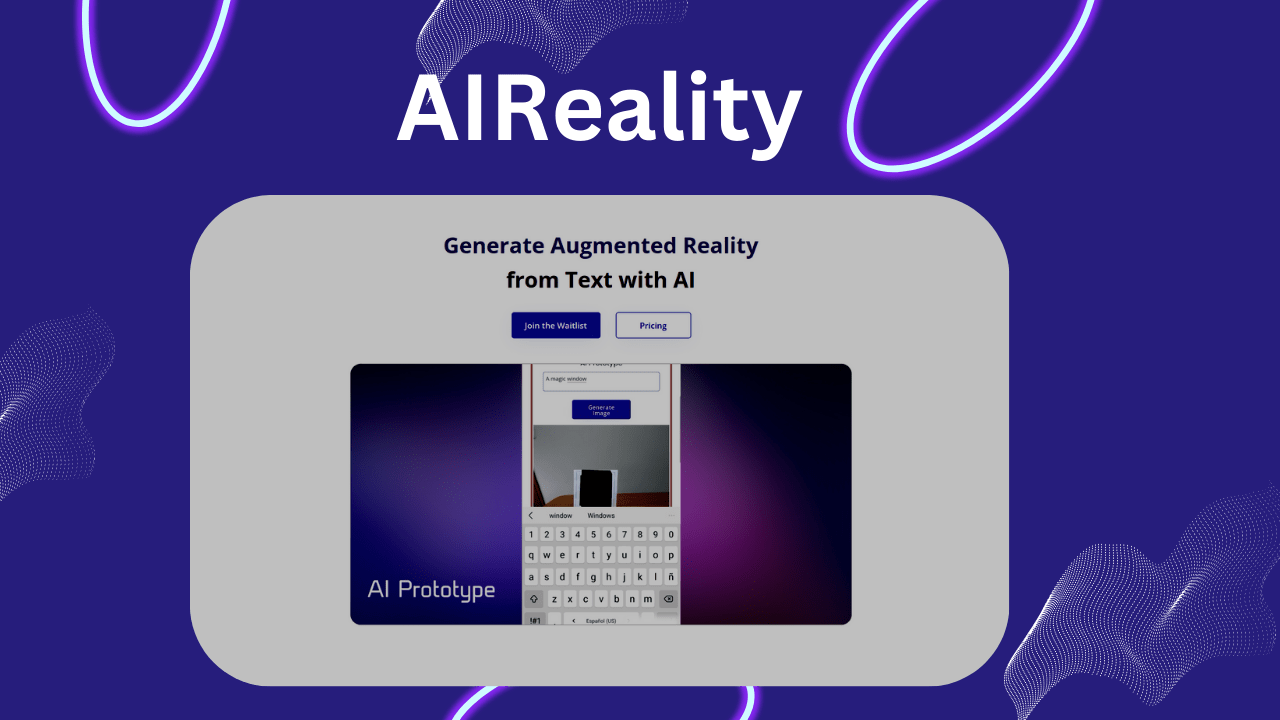
Visualizing the complex processes within Reality AI Lab requires clear and informative diagrams. These visualizations help researchers understand data flow, technology integration, and the impact of their work on various industries. The following sections detail illustrative examples of such visualizations.
Data Flow in a Reality AI Lab Simulation Pipeline
This visualization would be a flowchart, depicting the sequential stages of data processing within a typical Reality AI Lab simulation. The flowchart would begin with raw sensor data input (e.g., lidar point clouds, camera images, IMU data). Arrows would then indicate the flow to subsequent processing stages. These stages would include: data cleaning and preprocessing (noise reduction, outlier removal, data alignment); feature extraction (e.g., object detection, segmentation, classification); model training (using machine learning algorithms on processed data to create simulation models); simulation execution (running the models to predict outcomes); and finally, results analysis and visualization (outputting data in a user-friendly format like graphs or 3D models).
Different data types at each stage would be clearly labeled, such as point clouds, feature vectors, probability maps, and simulation results. The color-coding of the arrows could represent the type of data transformation happening at each stage. For example, green for data cleaning, blue for feature extraction, and red for model training.
Reality AI Lab focuses on cutting-edge AI applications, but sometimes even the most advanced researchers need a break! If you’re finding yourself needing a printer for those research papers, setting up the wifi on your Canon TS3420 might be the perfect distraction; check out this helpful guide for canon ts3420 wifi setup to get you back on track.
Once you’re done, you can jump back into the fascinating world of Reality AI Lab’s innovative projects.
Integration of AI Technologies in a Reality AI Lab Project
This illustration would show a system diagram. A central core would represent a specific Reality AI Lab project, perhaps focused on autonomous vehicle navigation. From this core, radiating outwards would be different AI technologies integrated into the project. These could include computer vision (for object detection and scene understanding), deep learning (for model training and prediction), reinforcement learning (for optimizing control strategies), natural language processing (for human-machine interaction), and robotics (for physical interaction with the environment).
Each technology would be represented by a distinct icon or block, with connecting lines illustrating the data flow and interaction between them. For example, the computer vision module would feed object detection data to the deep learning module for path planning. The diagram would highlight the synergistic relationship between these technologies, emphasizing how they collectively contribute to the success of the project.
The overall design would emphasize a clean, modular structure showcasing the independent yet collaborative nature of the integrated AI technologies.
Impact of Reality AI Lab Research on the Autonomous Driving Industry
This visualization could be a comparative bar chart. Two sets of bars would represent key performance indicators (KPIs) before and after the implementation of Reality AI Lab’s research in autonomous driving. KPIs could include: accident rates (reduction demonstrated by a shorter bar in the ‘after’ section), average driving time (shorter bar indicating improved efficiency), fuel consumption (shorter bar showing optimized fuel usage), and overall system reliability (taller bar showcasing improved reliability).
Reality AI Lab focuses on cutting-edge AI applications, and understanding real-world data is key. A great example of this is how telecom giants like bell mts use AI for network optimization and customer service. This kind of data-driven insight is exactly what Reality AI Lab aims to help companies leverage to improve their operations and gain a competitive edge.
The chart would clearly label each KPI and its corresponding values. A brief caption could explain that the reduction in accident rates is due to improved object detection and path planning algorithms developed by the Reality AI Lab, while the improved efficiency is a result of optimized route planning and traffic prediction models. The chart would visually demonstrate the positive impact of Reality AI Lab’s research on safety, efficiency, and cost-effectiveness within the autonomous driving industry, using quantifiable metrics.
Epilogue

Reality AI Lab represents a significant leap forward in our ability to simulate and understand the complexities of the real world. The ethical implications are considerable, demanding careful consideration as we harness this powerful technology. However, the potential benefits – from advancements in healthcare and engineering to improved urban planning and resource management – are transformative. The future of Reality AI Lab lies in continued innovation, interdisciplinary collaboration, and a commitment to responsible development, ensuring that this technology serves humanity’s best interests.
Detailed FAQs
What types of data does Reality AI Lab use?
Reality AI Lab utilizes a wide range of data, including sensor data, images, videos, and textual information, depending on the specific project and simulation requirements.
How does Reality AI Lab ensure the accuracy of its simulations?
Accuracy is ensured through rigorous validation processes, comparing simulation outputs with real-world data and incorporating feedback loops to refine models. The specific methods vary depending on the application.
What is the cost of developing a project using Reality AI Lab technology?
The cost varies significantly depending on project scope, complexity, and the required level of customization. A detailed cost analysis is provided on a case-by-case basis.
What kind of expertise is needed to work at Reality AI Lab?
Reality AI Lab requires a diverse team with expertise in AI, computer science, engineering, data science, and relevant domain expertise (e.g., healthcare, urban planning).